Generative AI for Hyper-Personalized Marketing Campaigns
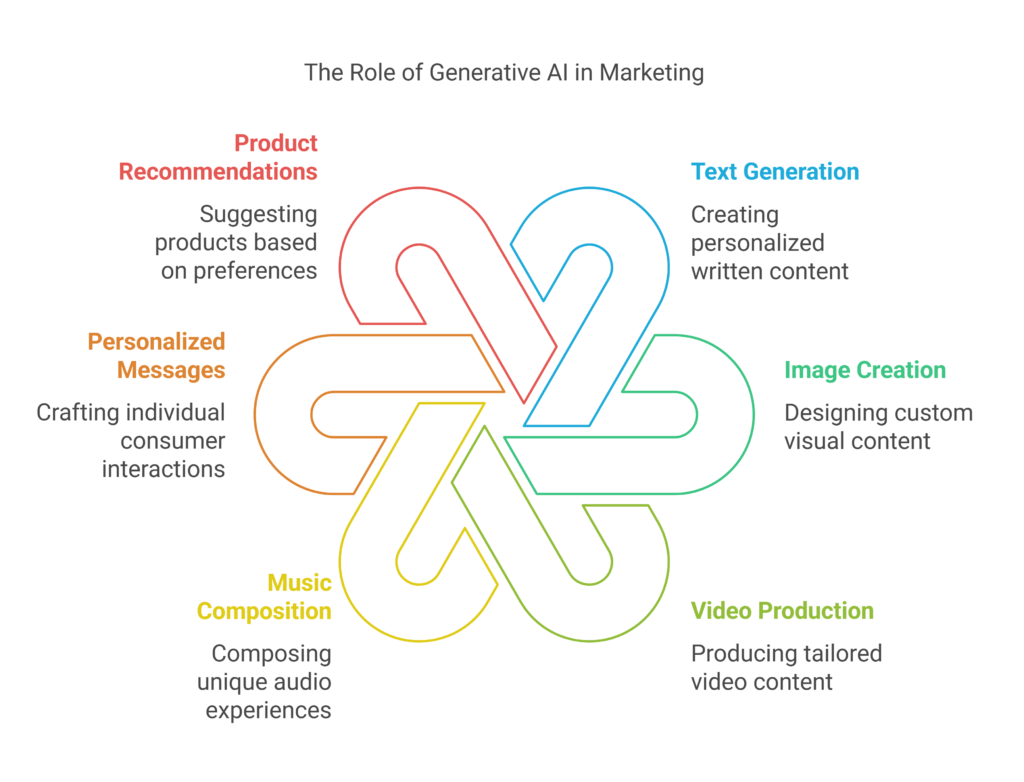
Introduction
Generative AI is a subset of artificial intelligence technology that may use patterns found in current data to create new material, including text, photos, videos, and even music. Because generative AI makes it possible to produce highly customised content at scale, it is completely changing the way brands engage with their audiences in marketing. By using tools like DALL·E, OpenAI’s GPT models, and others, marketers may create dynamic ads, personalised messages, and product recommendations that speak to specific customers.
Importance of Hyper-Personalization in Modern Digital Marketing
With the amount of content and ads that customers are exposed to in today’s digital world, it is becoming more and more difficult for companies to stand out. In order to overcome this difficulty, hyper-personalization provides material that is especially suited to each user’s tastes, habits, and requirements. Hyper-personalization uses real-time data and cutting-edge algorithms to offer each customer a unique experience, in contrast to traditional personalisation, which may divide audiences into broad categories. In addition to increasing engagement, this strategy cultivates trust and loyalty.
How AI-Driven Personalization Enhances Customer Experience and Conversion Rates
AI-driven personalisation goes beyond hyper-personalization by predicting consumer preferences and behaviours through the analysis of massive volumes of data. Generative AI, for instance, is capable of creating unique landing pages for each user, suggesting products based on browsing history, and crafting personalised email subject lines. AI improves the customer experience by providing timely and relevant content, which makes interactions seem more natural and significant. Higher conversion rates follow because consumers are more inclined to interact with and buy from companies that recognise and address their particular demands.
Understanding Generative AI
i. What is Generative AI?
The term “generative AI” describes a class of artificial intelligence systems that use patterns and structures found in existing data to create new content, such as text, photos, audio, or video. It makes extensive use of deep learning, a kind of machine learning that models complex data using multi-layered neural networks. Important elements of generative AI consist of:
- Natural Language Processing (NLP): Machines can now comprehend, interpret, and produce human language thanks to natural language processing, or NLP. Applications such as text generation, sentiment analysis, and chatbots are powered by NLP.
- Large Language Models (LLMs): It’s a sophisticated AI models that produce language that is contextually relevant and coherent after being trained on enormous datasets. The GPT (Generative Pre-trained Transformer) series from OpenAI is one example; it can write essays, respond to queries, and even produce code.
- Generative Adversarial Networks (GANs): A framework in which a discriminator and a generator neural network collaborate to produce lifelike pictures, movies, or other types of media.
ii. Evolution of AI in Marketing:
The use of AI in marketing has evolved significantly over the years:
- Rule-Based Systems: Early marketing automation tools relied on predefined rules to segment audiences and send generic messages. These systems were limited in flexibility and scalability.
- Predictive Analytics: With the advent of machine learning, marketers began using predictive models to analyze customer behavior and forecast trends. This allowed for more targeted campaigns but still lacked creativity.
- Generative AI: The latest evolution, generative AI, enables marketers to create dynamic, personalized content at scale. It combines data-driven insights with creative capabilities, allowing brands to craft unique experiences for each customer.
iii. Key AI Models Used in Marketing:
Several generative AI models have become indispensable tools for modern marketers:
- Generic Pre-trained Transformer (GPT): GPT models (such as GPT-3 and GPT-4) are LLMs created by OpenAI that can produce text that appears human. Applications include content production, chatbot interactions, ad copywriting, and customised email marketing.
- DALLE: OpenAI also created DALL·E, which uses textual descriptions to create visuals. Applications: Producing original images for advertisements, posts for social media, and product designs.
- Consistent Diffusion: An open-source generative AI model that uses text cues to produce high-quality images. Applications include creative storytelling, branding, and the creation of visual content.
- ChatGPT: A GPT-based conversational AI model intended for interactive discourse. Applications include lead qualification, customer service, and tailored suggestions.
- By Anthropic, Claude: A rival to GPT that focusses on producing morally and safely sound content. Applications include customer interaction, brand-safe messaging, and content moderation.
The Concept of Hyper-Personalization
i. Difference Between Personalization and Hyper-Personalization
Personalization
- Involves dividing up the consumer base into large groups according to their characteristics, tastes, or habits.
- Provides these groups with material that is tailored to their interests (e.g., “Recommended for You” depending on geography or age).
- Frequently makes use of predetermined rules and static data.
Hyper-Personalization
- Goes above and beyond segmentation to give every consumer a unique experience.
- Makes use of AI, machine learning, and real-time data to anticipate and address specific client needs.
- Provides extremely targeted content, such dynamic pricing, personalised product recommendations, or customised messaging depending on user behaviour in real time.
Data-Driven Marketing: How Customer Data Fuels AI Models
Large volumes of consumer data are needed to train and power AI models for hyper-personalization. In order to forecast consumer preferences, actions, and purpose, these models examine patterns and correlations in the data. Among the crucial elements of data-driven marketing are:
- Predictive analytics: AI algorithms estimate future behaviours, such purchase likelihood or churn risk, using past and current data.
- AI-powered real-time decision-making: It uses data processing to present tailored offers or information at the appropriate time (e.g., emailing a discount voucher when a buyer abandons their cart).
- Continuous Learning: As AI models digest more data, they get better at making predictions and suggestions.
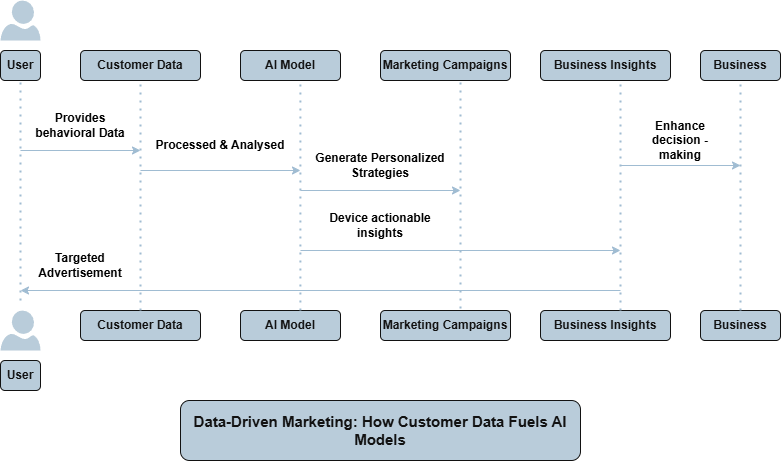
Sources of Data
Hyper-personalization depends on diverse data sources to build a comprehensive understanding of each customer. Key sources include:
- Behavioral Analytics:
- Tracks customer interactions with websites, apps, and emails (e.g., clicks, page views, time spent).
- Provides insights into customer intent and preferences in real time.
- CRM (Customer Relationship Management) Systems:
- Stores detailed customer profiles, including contact information, past interactions, and preferences.
- Helps marketers understand the customer journey and tailor communications accordingly.
- Purchase History:
- Records past transactions, including products bought, frequency, and spending patterns.
- Enables personalized product recommendations and loyalty programs.
- Social Media Activity:
- Monitors likes, shares, comments, and follows to gauge interests and sentiment.
- Helps brands engage customers with relevant content and campaigns.
- Third-Party Data:
- Includes demographic, geographic, and psychographic data from external sources.
- Enhances customer profiles and segmentation.
- IoT (Internet of Things) Data:
- Collects data from connected devices, such as smart home products or wearables.
- Provides real-time insights into customer behavior and preferences.
Use Cases of Generative AI in Marketing Campaigns
i. Dynamic Content Generation: AI-Generated Personalized Emails, Ad Copies, and Social Media Posts
Generative AI can create tailored content for individual customers based on their preferences, behaviors, and past interactions. Examples include:
- Personalized Emails: AI generates subject lines, body text, and calls-to-action that resonate with each recipient. For instance, an e-commerce brand might send an email with product recommendations based on browsing history.
- Ad Copies: AI crafts unique ad copy for different audience segments, optimizing for relevance and engagement.
- Social Media Posts: AI generates posts tailored to the interests of specific followers, increasing engagement and reach.
- Example: Tools like Jasper.ai or Copy.ai use GPT-based models to create marketing content that feels human-written and highly relevant.
ii. AI-Driven Visuals & Videos: Personalized Video Ads Using Generative AI
Generative AI can create custom visuals and videos tailored to individual customers. This includes:
- Personalized Video Ads: AI generates videos that incorporate a customer’s name, location, or past purchases. For example, a travel company might create a video showcasing destinations the customer has previously searched for.
- Dynamic Imagery: Tools like DALL·E or Stable Diffusion generate custom images for ads, social media, or product pages based on textual prompts.
- Example: Platforms like Synthesia or Pictory allow marketers to create personalized video content at scale without the need for expensive production teams.
ii. Conversational AI & Chatbots: AI-Powered Chatbots Delivering Tailored Responses
AI-powered chatbots and virtual assistants use natural language processing (NLP) to provide personalized customer support and recommendations. Key applications include:
- Customer Support: Chatbots answer queries, resolve issues, and guide customers through processes in real time.
- Personalized Recommendations: Chatbots suggest products or services based on customer preferences and past interactions.
- Lead Qualification: AI chatbots engage website visitors, ask qualifying questions, and route leads to the appropriate sales team.
- Example: ChatGPT-powered chatbots or Google’s Dialogflow enable brands to deliver seamless, personalized interactions across websites and messaging platforms.
iv. Automated Product Recommendations: AI-Curated Product Suggestions Based on Customer Behavior
Generative AI analyzes customer data to predict preferences and recommend products or services. This includes:
- E-Commerce Recommendations: AI suggests products based on browsing history, purchase behavior, and similar users’ preferences.
- Content Recommendations: Streaming platforms like Netflix or Spotify use AI to recommend movies, shows, or music tailored to individual tastes.
- Upselling and Cross-Selling: AI identifies opportunities to recommend complementary or premium products.
- Example: Amazon’s recommendation engine uses AI to drive a significant portion of its sales by suggesting relevant products to customers.
v. Real-Time Personalization in Websites & Apps: AI Modifying Website Content Dynamically
Generative AI enables websites and apps to adapt content in real time based on user behavior. This includes:
- Dynamic Website Content: AI changes headlines, images, or calls-to-action based on the visitor’s demographics, location, or browsing history.
- Personalized Landing Pages: AI creates unique landing pages for different audience segments or even individual users.
- Adaptive User Interfaces: Apps and websites adjust layouts, menus, or features to suit the preferences of each user.
- Example: Tools like Dynamic Yield or Optimizely use AI to deliver real-time personalization, improving user experience and conversion rates.
How Generative AI Works in Hyper-Personalization
Generative AI enables hyper-personalization by leveraging customer data to create tailored content and experiences. Below is a breakdown of the process:
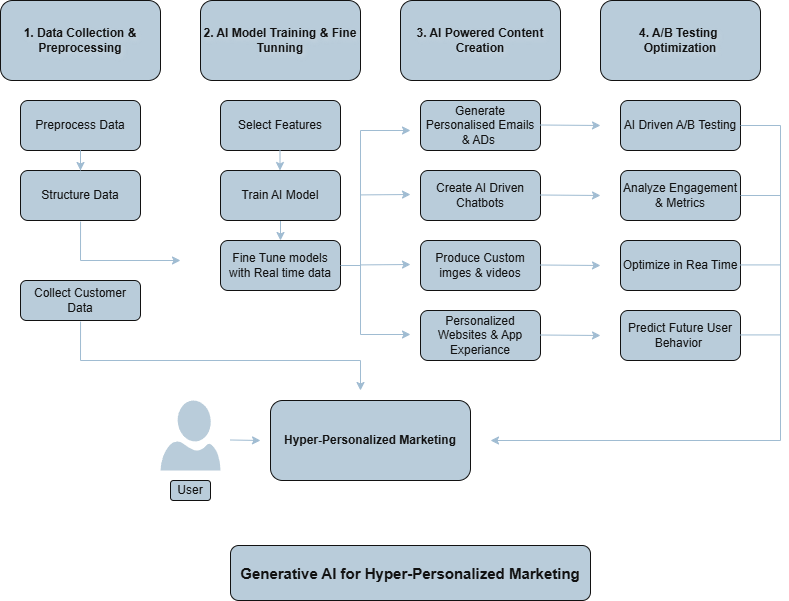
Technologies & Tools Powering AI-Driven Marketing
AI-driven marketing relies on a combination of advanced frameworks, platforms, and tools to deliver hyper-personalized and efficient campaigns. Below is an overview of the key technologies and tools:
AI Frameworks:
AI frameworks provide the foundation for building, training, and deploying machine learning models. Two of the most popular frameworks are:
i. TensorFlow:
- Developed by Google, TensorFlow is an open-source framework for machine learning and deep learning.
- Widely used for tasks like natural language processing (NLP), image recognition, and predictive analytics.
- Offers scalability and flexibility, making it suitable for both research and production environments.
ii. PyTorch:
- Developed by Facebook’s AI Research lab, PyTorch is another open-source framework known for its ease of use and dynamic computation capabilities.
- Popular in research and academia, PyTorch is increasingly used in production for tasks like generative AI and reinforcement learning.
AI Marketing Platforms
AI marketing platforms leverage generative AI to streamline content creation, personalization, and campaign management. Key platforms include:
i. ChatGPT (by OpenAI):
- A conversational AI model that generates human-like text for chatbots, email campaigns, and content creation.
- Applications: Customer support, personalized messaging, and ad copywriting.
ii. Jasper (formerly Jarvis):
- An AI-powered writing assistant that helps marketers create blog posts, social media content, and ad copy.
- Applications: Content marketing, SEO optimization, and email campaigns.
iii. Copy.ai:
- A generative AI tool for creating marketing copy, including product descriptions, social media posts, and email subject lines.
- Applications: E-commerce, social media marketing, and lead generation.
iv. Canva AI:
- Integrates AI tools into Canva’s design platform to automate tasks like image editing, background removal, and template suggestions.
- Applications: Visual content creation for ads, social media, and presentations.
iv. Midjourney:
- A generative AI tool for creating high-quality images and artwork from text prompts.
- Applications: Visual storytelling, ad creatives, and branding.
CRM & Data Analytics Tools (HubSpot, Salesforce, Google Analytics, Adobe Sensei)
Tools for data analytics and customer relationship management (CRM) are crucial for gathering, evaluating, and using consumer data to support AI-powered advertising campaigns. Important tools consist of:
i. HubSpot:
- A comprehensive CRM platform that integrates marketing, sales, and customer service tools.
- Features: Contact management, email marketing, and behavioral analytics.
- Applications: Lead nurturing, campaign tracking, and customer segmentation.
ii. Salesforce:
- A leading CRM platform with AI capabilities through its Einstein AI suite.
- Features: Predictive analytics, personalized recommendations, and automated workflows.
- Applications: Sales forecasting, customer engagement, and marketing automation.
iii. Google Analytics:
- A web analytics tool that tracks and reports website traffic and user behavior.
- Features: Audience insights, conversion tracking, and real-time analytics.
- Applications: Campaign performance analysis, customer journey mapping, and ROI measurement.
Future Trends & Innovations in Generative AI for Marketing
1. AI-Driven Autonomous Marketing Campaigns
The future of marketing lies in fully autonomous campaigns that leverage AI to plan, execute, and optimize without human intervention. Key features include:
- Self-Learning Systems: AI models will continuously learn from campaign performance, customer behavior, and market trends to refine strategies in real time.
- End-to-End Automation: From content creation to audience targeting and budget allocation, AI will handle every aspect of campaign management.
- Predictive Analytics: AI will predict customer needs and preferences, enabling proactive marketing strategies.
- Example: An AI system could automatically launch a holiday campaign, generate personalized content, adjust ad spend based on performance, and analyze results—all without human input.
2. AI-Powered Metaverse Advertising
Augmented reality (AR) and virtual reality (VR) are becoming powerful tools for immersive marketing. Generative AI will enhance these experiences by:
- Virtual Brand Experiences: AI will create interactive, immersive experiences in the metaverse, such as virtual stores, events, or games.
- Personalized Avatars: AI will generate custom avatars and virtual environments tailored to individual users.
- Dynamic Ad Placement: Ads will adapt in real time based on user behavior within the metaverse.
- Example: A fashion brand could use AI to create a virtual runway show where users’ avatars can try on and purchase digital clothing.
3. Generative AI in AR/VR Marketing Experiences
AI-powered chatbots and virtual assistants use natural language processing (NLP) to provide personalized customer support and recommendations. Key applications include:
- Creating Custom Content: AI will generate 3D models, animations, and interactive elements for AR/VR campaigns.
- Personalizing Experiences: AI will tailor AR/VR content to individual users, such as virtual try-ons for products or personalized virtual tours.
- Real-Time Adaptation: AI will modify AR/VR experiences based on user interactions and preferences.
- Example: A furniture brand could use AI to generate AR models of sofas that customers can visualize in their living rooms, with real-time adjustments for size, color, and style.
4. AI and Blockchain for Transparent Ad Personalization
The combination of AI and blockchain technology will address concerns around data privacy and transparency in personalized advertising:
- Data Security: Blockchain ensures that customer data is stored securely and used only with consent.
- Transparent Algorithms: AI models built on blockchain can provide transparency into how data is used for personalization, building trust with customers.
- Decentralized Advertising: Blockchain enables peer-to-peer ad networks, reducing reliance on centralized platforms and giving users more control over their data.
- Example: A blockchain-based ad platform could allow users to see exactly how their data is being used to personalize ads and even earn rewards for sharing their information.
Conclusion
Recap of Key Points
Generative AI is revolutionizing the marketing landscape by enabling hyper-personalized campaigns that deliver exceptional customer experiences. Key takeaways include:
- Generative AI leverages deep learning, NLP, and large language models to create dynamic, personalized content.
- Hyper-personalization goes beyond traditional personalization by using real-time data and AI to tailor experiences for individual customers.
- Use cases span dynamic content generation, AI-driven visuals, conversational AI, automated product recommendations, and real-time website personalization.
- Technologies and tools like TensorFlow, PyTorch, ChatGPT, Jasper, and CRM platforms power these innovations.
- Benefits include improved customer engagement, faster content creation, cost-effective campaigns, and increased ROI.
These advancements will enable marketers to deliver even more relevant, engaging, and memorable experiences, driving customer loyalty and business growth.
Final Thoughts on Leveraging AI Responsibly
While generative AI offers immense potential, it also comes with responsibilities. Marketers must prioritize:
- Data Privacy: Ensure customer data is collected, stored, and used ethically and transparently.
- Bias Mitigation: Regularly audit AI models to prevent biases that could lead to unfair or discriminatory outcomes.
- Human Oversight: Maintain a balance between automation and human creativity to ensure campaigns remain authentic and aligned with brand values.
- Sustainability: Consider the environmental impact of AI technologies and strive for energy-efficient solutions.
Generative AI is a game-changer for hyper-personalized marketing, offering unprecedented opportunities to engage customers in meaningful ways. As the technology continues to evolve, marketers who embrace it thoughtfully and ethically will lead the way in creating the future of customer experiences.